- Research Project
PPML-Health: a Privacy-Preserving Machine Learning Infrastructure for Health Research
The developed platform facilitates machine learning on data from over 400 million patients without taking patient-specific data out of its protected environment. This leads to improved diagnosis and treatment.
Factsheet
- Lead school(s) School of Engineering and Computer Science
- Strategic thematic field Institute for Cybersecurity and Engineering ICE
- Duration 01.02.2021 - 30.11.2022
- Project management Prof. Dr. Ulrich Fiedler
- Head of project Dr. Bernhard Bodenmann
-
Project staff
Dr. Andreas Walter (Deputy Project Manager)
Prof. Dr. Michael Krauthammer
Francesco Romeo
Taejun Moon -
Partner
Clinerion Ltd., Basel
Universität Zürich - Keywords Machine learning, federated learning, health data, data protection
Initial situation
The Patient Network Explorer (PNEx) software platform from data informatics company Clinerion provides real-time access to the electronic health records of over 400 million patients worldwide. This data is currently used primarily for the planning and optimisation of clinical trials, and for the recruitment of patients for these trials. However, there is still a wealth of untapped potential in the PNEx platform: with the help of machine learning, the data can be used for further analyses and even forecasts. However, existing cloud-based machine-learning solutions are not suitable for use in the healthcare sector due to the strict requirements with respect to patient data protection.
Procedure
A new approach was implemented within the PPML-Health project using what is known as ‘Federated Learning’, a type of machine learning in which sensitive data remains in the protected hospital environment. This is possible because in federated learning, models are trained simultaneously on multiple devices without the exchange of sensitive data. Only model parameters are shared at regular intervals with a central server, which supplies the models with new parameters based on the new information. This process uses ‘Federated Averaging’ and ‘Federated Proximity’ algorithms.
Bern University of Applied Sciences, the University of Zurich and Clinerion were involved in the project, which was funded by Innosuisse. Bern University of Applied Sciences was responsible for the development of the user interfaces and for building the machine-learning pipelines. The University of Zurich was responsible for developing the algorithms for Federated Learning. Clinerion was responsible for the implementation on their IT systems.
Results
The result of the project is the Federated Machine Learning Platform, officially launched by Clinerion in November 2022. This extension of the PNEx platform enables models to be developed that facilitate the personalised prediction of treatment outcomes, for example, or the discovery of symptom clusters. Personalised prediction helps doctors and insurance companies to better assess the efficacy and appropriateness of a proposed drug. The discovery of clusters helps pharmaceutical companies to find new effects and applications for existing drugs.
The developed models and the Federated Machine Learning Platform were installed in several hospitals in Clinerion’s IT infrastructure in the scope of the Innosuisse project. Installation in the remaining hospitals that are part of the PNEx network will take place over the next few years.
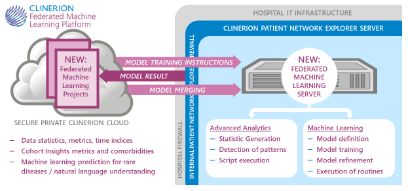
Perspective
Several clients of data informatics company Clinerion have already expressed interest in working with the platform to build models that predict patient journeys and to gain a better understanding of key factors at different points in the course of a treatment.
Clinerion clients are also interested in models to help doctors diagnose rare diseases by recommending screening for patients at high risk of developing a disease.